Aqualearning
Usando a Inteligência Artificial na gestão de recursos hídricos
Aqualearning
Voltar
Download the practice
People in charge of the innovative practice :
Ester Vilanova – ester.vilanova@amphos21.com
Aqualearning is a project created by Amphos21 and was the winner of the SmartCatalonia Challenge.
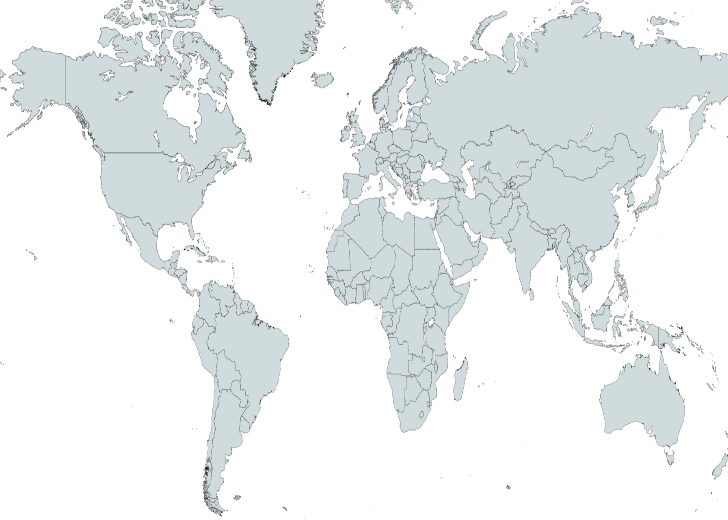
This environmental consultancy has developed a software based on artificial intelligence and machine learning that allows to value existing data, giving a new dimension to the processing of data and obtaining simulations at low computational cost. The application allows to find relationships-cause effect of the resources and natural systems applicable to the field of prediction of minority contaminants, control of well levels, f…
With the development of sensors, the increase of data volumes and their great generation, these tools facilitate data processing, efficient management and decision making, avoiding the use of resources, reducing costs and time.
Responsible entity
Amphos 21 is a scientific, technical and strategic consulting company at the service of the environment in 5 main markets (nuclear, mining, water, sustainability and CCS and climate change) and economic and social development. Amphos 21 began its activity in Barcelona in 1994. Currently the company includes more than 200 professionals, the 25 years of experience as an international consultant have allowed to develop projects in more than 20 countries. (CWP, 2022).
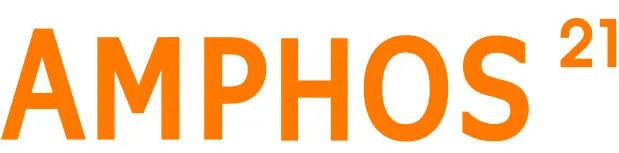
Recuperado de: https://www.amphos21.com/
The focus of the firm Ampos21 is to propose efficient, innovative, integrated and transversal solutions applying a quantitative method to any solution. The consultancy has acquired extensive experience in the evaluation, analysis and modelling of a wide range of processes in geological media, including hydrogeology, geochemistry, geomechanics and coupled reactive transport. In this context they visualize hydrological resources as an integrated solution, which synthesizes all relevant aspects of the hydrological cycle for a given project.
Detailed explanation
Aqualearning is a solution based on artificial intelligence (AI) supported by IML’s own software. Its main function is to transform data into valuable information. The process followed can be broken down into the following fields:
In the first place, the analysis and preparation of the data, evaluating the variables, finding correlations, discriminating the data that provide information from those that do not.
A part of the data is used to ‘train’ the algorithm. In this sense, once the algorithm is ‘trained’, its predictions are validated. Validation uses unused data to see if it has the ability and robustness to generate predictions as accurately as possible. When the predictions are already reliable, the algorithm is prepared to generate future alerts or decision making. Aqualearning contains different algorithms developed using open source (TensorFlow technology).
Aqualearning benefits from the cause-effect relationship inherent in natural resource-related processes to manage them using AI.
The software was applied in different fields:
First, for the prediction of minority elements in the aquifer of Forsmark (Sweden) where it is planned to build a deep store of nuclear waste. From physicochemical data and majority elements (collected since 1994), by means of a decision tree (algorithm typology) it was possible to correlate the appearance of minority elements with the presence of some majority elements. The hypothesis was correct, with a high coefficient of determination and allowed to predict the concentrations of barium (97% average accuracy) and uranium and molybdenum (>85% average accuracy).
It was also applied to the water resource affected by mining in Chile and Peru. In these countries, a part of the mining waste generates effluents of strong acidifiers. Currently the experimental process to evaluate the effect of the pH of the waters of each new exploitation, or expansion of this on the environment is slow (30 weeks). From the data of the first weeks and the concentrations of some elements (copper), the Aqualearning software is able to predict the effect of the pH of the waters at the end of the experiment. The result was achieved with more than 90% accuracy (using 4230 data), while copper concentrations (85% accuracy) were predicted. This contribution makes it possible to speed up the sizing of mine treatment plants in Peru in advance. Although it does not replace the experimental process, the results of Aqualearning have been a great help in the planning of actions and investments in the cases tested.
Finally, another application of this predictive methodology was made in a groundwater pumping field with 14 active wells. It is known that some of them interfere, but due to the geology and the arrangement of the fractures it is not identified which of them they are. Using Aqualearning it was possible to predict the levels of the wells by means of the flow data extracted during the previous days, obtaining a satisfactory adjustment (ICRA, 2022), (IDAEA, 2022).
Institutional setting
Aqualearning es un proyecto interno de Amphos21 que cuenta con financiación propia. Es el proyecto ganador del SmartCatalonia Challenge, competition promoted by “Polítiques Digitals i Administració Pública” of “Generalitat de Catalunya” (ACA, 2022).
Geographical setting
The project does not have a defined location, activities have been carried out locally (Catalonia), Europe (Sweden) and in Latin America (Chile and Peru).
Historical overview
In the mid-twentieth century, the technologies of control of water resources exploitations were manual, requiring a minimum knowledge and the transfer of this. Subsequently, the alert systems enabled the entities of the sector to reduce personnel costs and increase their efficiency.
With the advent of telecommunications, it was possible to remotely sensorize and monitor the collection facilities to collect the necessary data for management and decision making. (R. Lopez, 2020)
Recently, advanced software analyzes data in order to acquire valuable information in real time. The current challenge is to use the data in the prediction of the system’s responses to a given situation based on the information previously collected (big data).
Evidence of benefits from implementation
The application of the Aqualearning software values series of historical data, extracting from them relevant information for the management of any conveniently monitored system. These tools streamline, reduce costs and allow prediction and early warning with criteria, for example, in the sustainable management of aquifers. It is, therefore, in this case, a quantitative error of decision-making for the optimization of the exploitation of wells. Historical data is used to characterize aquifer response and catchment performance, control contaminant concentration, or reduce energy costs.
It has, therefore, a relevant application in the optimization of the water supply service, currently consisting of a completely innovative approach for which Aqualearning supposes a technical and methodological positioning of reference.
Replication potential in SUDOE region
This application is replicable in any system of exploitation of the hydrogeological resources of the SUDOE region that has a data acquisition system, and for which predicting a cause-effect relationship using AI can provide an improvement in the management of the activities developed.
Future outlook
There is no defined limit and it is applicable in many fields, including water resources management. Constant data collection is already part of the management action. Parallel to the use of sensors, the different decision-making technologies based on the data collected are constantly evolving. The use of AI is postulated as a methodology of wide possibilities.
However, it will be necessary to improve the data processing process, the homogenization of these and develop the analysis capabilities for the use of AI in the optimization of tasks and decision making.
Key points of the innovative method
- Aqualearning se posiciona como una herramienta pionera para la aplicación de la IA para la gestión de la explotación de recursos hídricos.
- Es una herramienta eficiente y sus aplicaciones en diversos casos han sido exitosas.
Acknowledgements
Innovative practice was suggested por Ester Vilanova Muset of the company AMPHOS 21.
References
- Agència Catalana de l’Aigua. (s/f).SmartCatalonia Challenge. Recuperado el 7 de marzo de 2022, de https://aca.gencat.cat/ca/actualitat/smart-catalonia-challenge
- Institut Català de Recerca de l’Aigua (ICRA) [InstitutCatalàdeRecercadelAiguaICRAGirona]. (2022, marzo 22). World Water Day 2022: Aigua subterrània: Fent l’invisible, visible. Youtube. https://www.youtube.com/watch?v=rflV1eQuX9Q
- IDAEA-CSIC [IDAEA-CSIC]. (2022, abril 5). WATER TALKS – Reptes i solucions per a la gestió i tractament d’aigües subterrànies. Youtube. https://www.youtube.com/watch?v=cDJ5fCyuiX8
- López, R. (2020, noviembre 5). Compuerta 4.0, historia de la transformación digital. iAgua. https://www.iagua.es/blogs/ramon-lopez/compuerta-40-historia-transformacion-digital
aquifer
NOTÍCIAS
Descubra mais sobre as notícias do projeto AQUIFER e sobre a gestão de aquíferos
NOTÍCIAS AQUIFER
Descrição e objectivos do projecto
The scientific community recommends a substantial improvement in the knowledge of aquifers, the establishment of reliable monitoring networks and a greater involvement of the administration and users to achieve a sustainable management of aquifers. The main objective...
Informação sobre o projecto
A Comunidade de Utilizadores de Água do Delta Llobregat concebeu bacias de recarga em Molins de Rei para recarregar o aquífero do Baix Llobregat. Vista de uma das bacias de recarga durante a fase de teste A Comunidade de Utilizadores de Água de Llobregat Delta é um...
Histórias de sucesso na gestão das águas subterrâneas
Compilação de histórias de sucesso na gestão das águas subterrâneas. Ao longo do mês de Abril, os 30 casos de práticas inovadoras na gestão das águas subterrâneas já foram seleccionados pelos agrupamentos que participam no projecto: PPA, CWP e AV. A tarefa começou com...
PROPONHA UMA
PRÁTICA INOVADORA
Está a desenvolver ou implementar uma prática inovadora em matéria de gestão de aquíferos e deseja referência-la na plataforma do projeto AQUIFER?
Preencha o formulário e faça uma proposta aos parceiros do projeto AQUIFER.
THE E-BOOK
O Aquífero oferece uma gama de práticas inovadoras de gestão da água. Pode descarregar todas as nossas fichas técnicas aqui.
E-BOOK DE PRÁTICAS INOVADORAS
DOCUMENTAÇÃO
Aprofunde a informação relacionada com a gestão dos aquíferos